January 5, 2017
At the 2016 meeting of the Biodiversity Information Standards organization (TDWG), Matthew Collins, Jen Hammock (Encycopedia of Life), and Alexander Thompson chaired a symposium titled “Big Data Analysis Methods and Techniques as Applied to Biocollections”. The TDWG community is at a turning point that is a reflection of the success of the work that they have done over the past decades. Large biodiversity datasets and the computing resources to use them are now commonly and freely available and the community is ready to start doing analyses that cross taxonomy, space, genetics, and time. This symposium was an opportunity to bring those kinds of analyses in front of the whole TDWG group.
As an introduction to big data analysis, this symposium had a great collection of talks. Two talks, “Clustering botanical collections data with a minimised set of features drawn from aggregated specimen data” by Nicky Nicholson from the Royal Botanic Gardens and “Large-scale Evaluation of Multimedia Analysis Techniques for the Monitoring of Biodiversity” by Alexis Joly from INRIA Sophia-Antipolis introduced the value of two critical data science concepts: clustering and classification. These are the basic goals of big data analysis: find groups of similar things in a dataset without knowing the criteria for similarity and conversely if you can identify what group a bunch of things should be in, then learn how to assign new things to groups automatically. Both presenters did a great job of providing a feel for how these techniques work and what kinds of results can be obtained from each.
Steven Kelling from the Cornell Lab of Ornithology presented a talk titled “Taking a Big Data Approach to Estimating Species Abundance” which provided a higher-level description of the challenges and costs of analyzing large datasets with computational models. The eBird project has addressed the computational scale issues by buying resources in Microsoft’s Azure cloud which has reduced the time it takes to make species distribution visualizations down from days to hours but it still means that each visualization has a cost in the range of hundreds of dollars.
The last three talks related to how having an infrastructure for analyzing big data might affect the field. While it is important to introduce data science concepts to TDWG, it is equally important to reduce the barriers to working with the large and heterogeneous datasets required to apply them. As Dr. Kelling pointed out, this type of science currently requires technical engineering and money.
Matthew Collins presented “GUODA: A Unified Platform for Large-Scale Computational Research on Open-Access Biodiversity Data”. GUODA is a collaboration that brings expertise and infrastructure around Apache Spark and large data sets to the biodiversity community. These capabilities must be provided to the scientists directly and not something only available through software developers. To do that, a beta Jupyter Notebook server has been set up at http://jupyter.idigbio.org to let anyone start writing Spark code in Python or R to process datasets from iDigBio, GBIF, and the Biodiversity Heritage Library (BHL).
Jen Hammock from the Encyclopedia of Life (EOL) gave a talk titled “Fresh Data: what's new and what's interesting?”. She presented a web application called Fresh Data (http://gimmefreshdata.github.io/) which generates notifications of new species observations based on this infrastructure. This is something not possible without using both big data processing techniques and a re-envisioning of data sources not as silos that sit with individual projects but as resources to be aggregated and processed in real time. This ability to do responsive processing on data in place is important for the community to move into the big data era.
The final talk by Alexander Thompson title "Data Quality at Scale: Bridging the Gap between Datum and Data" covered the integration between the concepts discussed earlier in the conference during the data quality sessions and the idea of big data workflows. Data quality is a good application of these workflows because the individual measures of quality are weak and quality is often relative to the rest of the data for measures like outliers and clustering. By using clustering and classification techniques as part of the process of bringing the data into data aggregators, data can be improved beyond what a single provider can do on their own .
Thank you to all of our presenters for making this a great session. The symposium (and nearly all others at TDWG 2016) were recorded. You can watch videos of talks you missed and read more about iDigBio's participation at TDWG 2016 at https://www.idigbio.org/wiki/index.php/TDWG_2016_Annual_Conference . Direct links to each talk hosted in iDigBio's Vimeo channel:
- Clustering botanical collections data with a minimised set of features drawn from aggregated specimen data
- Taking a Big Data Approach to Estimating Species Abundance
- Large-scale Evaluation of Multimedia Analysis Techniques for the Monitoring of Biodiversity
- GUODA: A Unified Platform for Large-Scale Computational Research on Open-Access Biodiversity Data
- Fresh Data: what's new and what's interesting?
- Data Quality at Scale: Bridging the Gap between Datum and Data
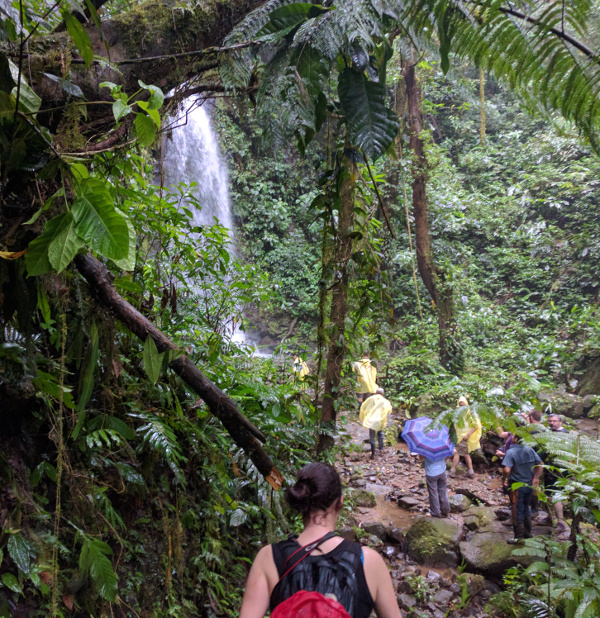